Cognitive K.i. Empowering AI Solutions for Professionals in Diverse Fields
Cognitive K.i.
Cognitive K.i. represents a significant advancement in the synthesis of artificial intelligence, blockchain technologies, and data science, developed by Black Cactus. This innovative platform is designed to harness AI's potential to facilitate intelligent decision-making processes while ensuring the integrity and security of data through blockchain. By integrating these technologies, Cognitive K.i. Offers a robust framework that addresses modern complexities in various sectors, including finance, healthcare, and supply chain management.
The intersection of AI and blockchain provides a dual advantage: AI algorithms analyze large datasets, identifying patterns and generating insights, while blockchain technology guarantees transparency and immutability. This combination not only enhances data reliability but also fosters trust among stakeholders. Furthermore, the use of data science methodologies amplifies the ability of Cognitive K.i. to generate predictive models, enabling organizations to anticipate trends and respond proactively.
As industries increasingly grapple with data-driven challenges, Cognitive K.i. positions itself as a cutting-edge solution that promotes efficiency and innovation. The platform’s capabilities underscore the transformative potential of merging artificial intelligence with blockchain, paving the way for smarter, more secure applications in an ever-evolving digital landscape. Thus, Cognitive K.i. signifies a pivotal step forward in the realm of integrated technological solutions.
Cognitive Neural Networks in Cognitive K.I
Cognitive K.I., which employs a neural network architecture, leverages synthetic data to mimic human cognitive processes. At the core of this paradigm are neurons, the fundamental units that receive input, process it, and generate output, analogous to the biological neurons within the human brain. These neurons are organized into layers: an input layer that receives data, one or more hidden layers responsible for processing, and an output layer that delivers results.
The connections between these neurons possess weights that indicate the strength of interaction. During the training phase, the neural network adjusts these weights based on the input from the synthetic data, enhancing its ability to discern patterns and make accurate predictions. This learning process is facilitated by the application of activation functions, which determine each neuron's output based on its input.
Cognitive K.I.'s reliance on synthetic data allows for exploring scenarios that might be impractical or unethical to study in reality and enables the model to generalize beyond the specific examples seen during training. Thus, cognitive K.I.'s cognitive function reflects an advanced synthesis of computational techniques, ultimately contributing to artificial intelligence's capacity for problem-solving and decision-making in complex environments
K function in Cognitive K.i.
The "K function" in data science and mathematics encompasses two pivotal concepts: the K-function in spatial statistics and K-means clustering in machine learning. The K-function serves as an essential spatial analysis tool to evaluate the clustering or dispersion of points within a specified area. By calculating the expected number of additional points within a particular distance from a randomly selected point, the K-function allows researchers to discern whether a spatial pattern is clustered, dispersed, or follows a random distribution. This analysis is often visualized through plots of K(r) values against distance (r), elucidating the nature of clustering or dispersion across various scales.
Conversely, K-means clustering is a widely employed unsupervised machine learning algorithm designed to partition data points into clusters. This algorithm operates by iteratively assigning each data point to the nearest cluster centroid, subsequently recalibrating the centroid positions as the mean of the assigned points. This process persists until cluster assignments stabilize, thus organizing data points into distinct groups. The user predetermines the number of clusters, and the algorithm seeks to minimize the within-cluster variance, ensuring that points within the same cluster are as similar as possible. Together, these concepts illustrate the diverse applications of the "K function," highlighting its significance in spatial analysis and data clustering.
(i) maginary numbers in Cognitive K.i.
Imaginary and complex numbers enrich various mathematical disciplines, offering essential tools in engineering, physics, and signal processing. Their significance extends beyond theoretical frameworks, providing a robust foundation for innovations in artificial intelligence, where complex algorithms often rely on these mathematical constructs. Thus, imaginary numbers are pivotal in advancing both mathematics and technology.
Imaginary numbers, integral to advanced mathematics, emerge from the necessity to address equations that do not possess real-number solutions, most notably exemplified by the equation (x^2 + 1 = 0). The concept of the imaginary unit (i), defined as the square root of (-1), opens the door to a broader mathematical framework. An imaginary number is conventionally expressed as (bi), where (b) is a non-zero real number. Notable examples include (3i), (-2i), and (frac{1}{2}i).
Algebraically, squaring an imaginary number (bi) yields (-b^2), as derived from the fundamental property (i^2 = -1). For instance, ((3i)^2 = 9i^2 = 9(-1) = -9). The synthesis of imaginary numbers with real numbers gives rise to complex numbers, articulated in the standard form (a + bi), where (a) represents the real component, and (bi) signifies the imaginary part. An example, (2 + 3i), illustrates this crucial interplay.
Cognitive K.i
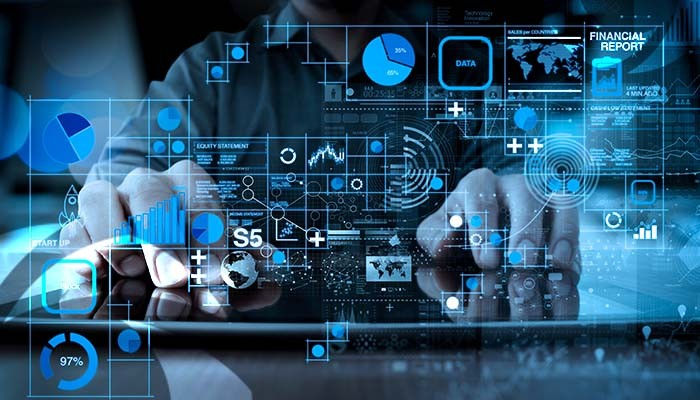
Data science