Cognitive K.i. Empowering AI Solutions for Professionals in Diverse Fields
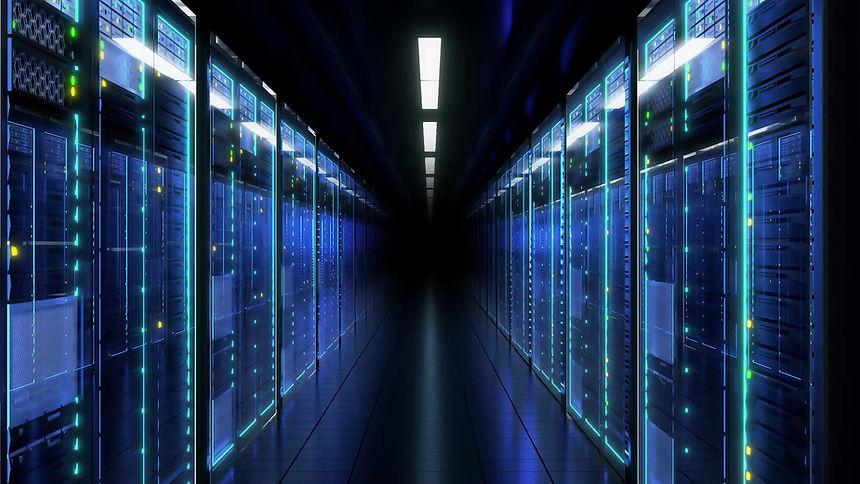
Data Science
Data science is a multidisciplinary field that combines computer science, statistics, mathematics, and domain expertise to extract actionable insights from data. It involves collecting, cleaning, analyzing, and visualizing data to uncover patterns, trends, and insights that can inform decision-making and improve processes.
Data Science
k.i. - Data Science
Data science, a multidisciplinary domain that employs scientific methods, algorithms, processes, and systems to extract knowledge and insights from structured and unstructured data, plays a pivotal role in the advancements of artificial intelligence (AI). The synergy between data science and AI is crucial, as data serves as the foundational element from which AI systems learn, adapt, and evolve. Data science effectively transforms raw data into actionable intelligence through a confluence of various activities such as data mining, data cleaning, and the utilization of data lakes and housing solutions.
The Role of Data in AI
At its core, artificial intelligence relies heavily on data. Machine learning (ML), a prominent subset of AI, requires vast datasets for training algorithms to recognize patterns. These datasets can be derived from multiple sources, commonly categorized into "big data" and "small data." Big data refers to large datasets that cannot be easily managed using traditional data processing applications. It is characterized by the volume, velocity, and variety of data generated, such as vast social media datasets or real-time transactional information from financial institutions.
In contrast, small data encompasses manageable datasets that can be easily analyzed and require less sophisticated techniques. While both data types are essential for different applications, their treatment and analysis largely depend on the methods employed in data science practices.
Data Lakes and Data Housing
Data lakes and data housing represent critical components in maintaining the integrity and accessibility of data. A data lake is a centralized repository that allows organizations to store raw data in its native format until it is needed for analytics. Unlike traditional databases that require data to be structured before storage, data lakes embrace unstructured, semi-structured, and structured data, making them versatile for big data applications.
On the other hand, data housing refers to the architecture and framework that supports data storage and management within an organization, which includes Data Warehouses and other storage solutions. Data housing typically involves structured data storage, making it easier for analysts to run complex queries and generate insights.
Data Cleaning and Preparation
Data cleaning, often seen as one of the most arduous tasks in data science, involves improving data quality by identifying and rectifying inaccuracies or inconsistencies within the data. Dirty data can lead to erroneous insights and decisions in AI applications, underscoring the importance of this process. Through techniques such as deduplication, normalization, and correction of missing values, data scientists prepare datasets for ensuing analysis.
Data preparation goes hand-in-hand with data cleaning. Before data can fuel machine learning models, it must be adequately formatted, chosen, and sometimes augmented with additional relevant data. This stage of the data science process acts as a foundation for successful AI applications.
Data Mining
Data mining, another critical process in data science, encompasses extracting patterns and knowledge from large volumes of data using statistical and computational techniques. By employing algorithms designed for clustering, classification, regression, and association analysis, data mining enables organizations to uncover meaningful insights hidden within immense datasets. This is particularly beneficial for AI, as data mining algorithms facilitate developing and training effective and robust models that can predict outcomes and inform decision-making.
Integration with Artificial Intelligence
Integrating data science techniques with AI culminates in training machine learning models and their deployment into real-world applications. Data scientists leverage the processed and cleaned data to train algorithms that perform natural language processing, image recognition, and predictive analytics.
Once trained, these models can improve over time with new data, honing their ability to make accurate predictions and decisions. This capability becomes particularly profound when harnessed in systems such as recommendation engines, autonomous vehicles, and healthcare diagnostics, where data-driven insights can lead to meaningful improvements in efficiency and effectiveness.