Cognitive K.i. Empowering AI Solutions for Professionals in Diverse Fields
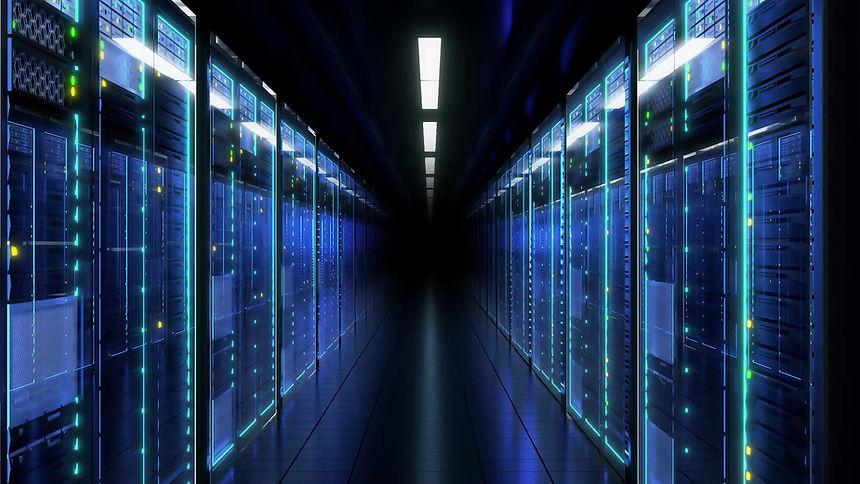
Data Mining
Data mining is the process of sorting through large data sets to identify patterns and relationships that can help solve business problems through data analysis. Data mining techniques and tools help enterprises to predict future trends and make more informed business decisions
Data Mining
k.i. - Data mining
Data mining is a multifaceted process that entails discovering patterns and knowledge from large datasets utilizing various analytical methods. It bridges the disciplines of statistics, machine learning, database management, and data visualization, presenting practitioners with a rich array of tools and techniques to derive valuable insights from voluminous quantities of information. As the digital age continues to burgeon, the significance of data mining in facilitating informed decision-making has become increasingly evident across diverse sectors, including healthcare, finance, marketing, and social sciences.
Data mining involves several essential steps. The initial stage is data collection, where relevant data is gathered from various sources, such as databases, data warehouses, and the Internet. This is followed by data preprocessing, which includes cleaning the data to remove inconsistencies and redundancies and transforming it into a suitable format for analysis. This stage is crucial, as the effectiveness of the subsequent mining process is inherently dependent on the quality and integrity of the data used.
Once the data has been prepared, the actual mining process commences. This involves applying various algorithms and models to extract meaningful information. Standard techniques employed in data mining include classification, clustering, regression, and association rule learning. Classification seeks to assign data points to predefined categories based on their attributes, making it invaluable for spam detection or credit scoring applications. Clustering, contrastingly, groups similar data points without prior knowledge of categories, serving to identify inherent structures within the data, as seen in customer segmentation analyses.
Regression analysis attempts to predict a continuous outcome variable based on one or more predictor variables. This proves particularly useful in forecasting sales or evaluating risk factors in medical studies. As exemplified by market basket analysis, association rule learning investigates relationships between variables, identifying sets of products often purchased together, thereby guiding inventory management and promotional strategies.
Advances in computing power and the proliferation of big data technologies have catalyzed the evolution of data mining, enabling the more efficient analysis of large and complex datasets. Tools such as Apache Hadoop and Spark facilitate distributed processing, offering the scalability and speed required to handle vast amounts of real-time data. These technological advancements have made it feasible for organizations to adopt data mining techniques on a larger scale, thus enhancing their ability to generate insights and derive strategic advantage.
The ethical implications surrounding data mining have gained considerable attention in conjunction with technical methodologies. Aggregating and analyzing personal data raises concerns about privacy, consent, and the potential misuse of information. As organizations increasingly leverage data mining to inform policy decisions, market strategies, and customer profiling, the need for establishing ethical frameworks and regulatory guidelines becomes paramount. Data protection laws, such as the General Data Protection Regulation (GDPR) in the European Union, emphasize the importance of safeguarding individual privacy and ensuring responsible data usage.
Data mining applications are extensive and varied, cutting across myriad domains. In healthcare, data mining techniques enable predictive analytics that can identify disease outbreaks, improve patient care, and optimize operational efficiency. In finance, organizations employ data mining to detect fraud, assess credit risk, and tailor financial products to meet consumer needs. Retailers utilize data mining to understand purchasing behavior, personalize marketing efforts, and manage inventory effectively. Social media analysts harness data mining to gauge public sentiment, track trends, and assess the impact of campaigns.
The intersection of data mining and artificial intelligence (AI) is noteworthy, as machine learning algorithms drive significant advancements in the field. With the ability to learn from data patterns and improve over time, AI-enhanced data mining techniques have revolutionized predictive analytics and automated decision-making processes. As these technologies continue to mature, they promise to transform how organizations interact with data and respond to emerging challenges.