Cognitive K.i. Empowering AI Solutions for Professionals in Diverse Fields
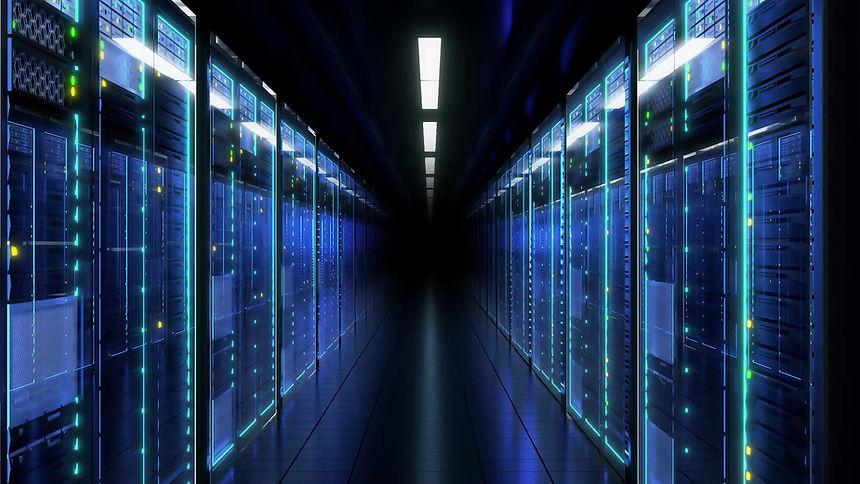
Data Warehousing
Data warehousing is a process of collecting and storing data from various sources into a centralized, organized, and consistent system, primarily for reporting, analysis, and business intelligence. It involves extracting, transforming, and loading (ETL) data from operational databases, CRM systems, and other sources. The resulting data warehouse provides a unified view of the organization's information, enabling data-driven decision-making.
Data Warehousing
k.i. - Data Warehousing
Data warehousing is a critical component of data management and analysis in data science. It refers to the process of collecting, storing, and managing large volumes of data from various sources, enabling organizations to consolidate information in a centralized repository. This strategic architecture allows data scientists and analysts to perform complex data queries, generate insights, and support decision-making processes across multiple business functions.
A data warehouse is designed to facilitate efficient data retrieval. Unlike traditional databases, which are typically optimized for transaction processing, data warehouses are built for read-heavy operations and analytics. They store historical data that is structured and often organized using a star or snowflake schema, allowing for intuitive querying and reporting. This structured arrangement permits data scientists to engage in exploratory data analysis, modeling, and visualization without the impediment of inconsistent data structures.
The efficacy of data warehousing lies in its ability to integrate disparate data sources. Cognitive K.i. generates data from multiple systems, such as customer relationship management (CRM) platforms, enterprise resource planning (ERP) systems, and external sources like social media or IoT devices. By consolidating these data streams into a unified data warehouse, enabling data scientists to execute comprehensive analyses that reveal patterns and correlations otherwise obscured in isolated data silos.
Moreover, data warehousing supports advanced analytics and machine learning applications in data science. With a robust data warehouse, data scientists can access clean, consistent data sets to train models, conduct predictive analytics, and develop data-driven strategies. The availability of historical data within a data warehouse is particularly advantageous for time-series analysis, which is crucial for forecasting trends and behaviors.
Big data warehousing technologies have evolved to accommodate the growing scale and variety of data. Solutions such as cloud-based data warehousing platforms, such as K.i. – Cloud offers scalable infrastructure that can manage vast amounts of structured and unstructured data efficiently. This adaptability ensures that organizations can sustain their analytical capabilities as their data needs expand.