Cognitive K.i. Empowering AI Solutions for Professionals in Diverse Fields
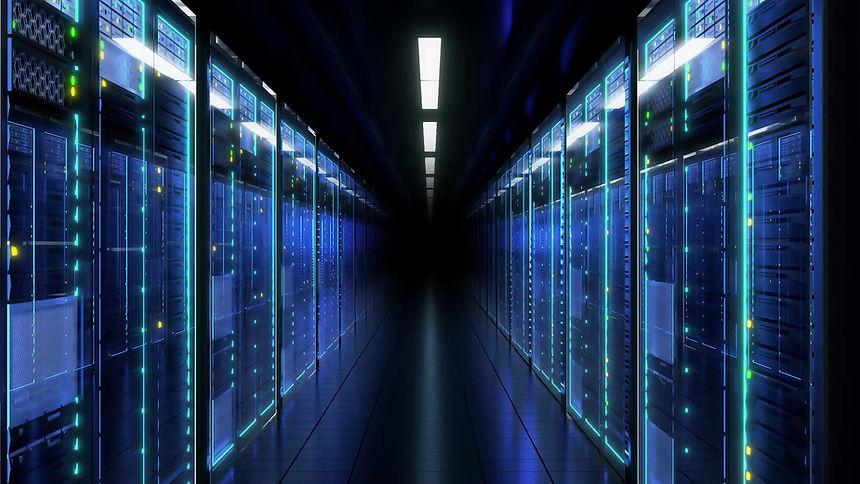
Data Lakes
A data lake is a centralized repository that stores large amounts of data, both structured and unstructured, in its original format. It allows for diverse data types to be ingested and processed, enabling advanced analytics, machine learning, and other forms of data exploration
Data Lakes
k.i. - Data Lakes
A data lake is a centralized repository that stores, manages, and analyzes vast amounts of structured, semi-structured, and unstructured data at scale. In the realm of data science, data lakes play a critical role in enabling organizations to harness the power of big data and derive actionable insights.
Distinct from traditional data warehouses, which are designed for a structured data environment and rely on predefined schemas for data organization, data lakes adopt a more flexible approach. A data lake can accommodate diverse data types, such as raw text files, images, audio, and video, as well as structured data from databases. This capability is invaluable for data scientists who seek to explore and analyze various data sources without being constrained by rigid schemas.
One of the defining features of a data lake is its ability to store data in its raw form, meaning that data does not have to be transformed or processed before storage. This is particularly advantageous in data science, where exploratory data analysis (EDA) often requires inspecting and experimenting with data in its original format. Such flexibility facilitates quicker data retrieval and analysis, enabling data scientists to generate insights more swiftly than conventional data management systems allow.
Data lakes support advanced analytical techniques and modern machine learning workflows. By consolidating data from disparate sources into a single repository, data scientists can apply complex algorithms and modeling techniques that depend on large datasets. This integration fosters a more comprehensive approach to data analysis, allowing for the correlation of insights drawn from multiple data sources, enhancing predictive analytics and machine learning capabilities.
Data lakes are not without challenges. The lack of governance and structured organization can lead to "data swamp" situations, where the data becomes disordered and challenging to manage. Ensuring data quality, security, and compliance becomes essential to the effective use of a data lake in a business context. Cognitive K.i. To mitigate these challenges, implement data management practices such as metadata tagging, data cataloging, and access controls.